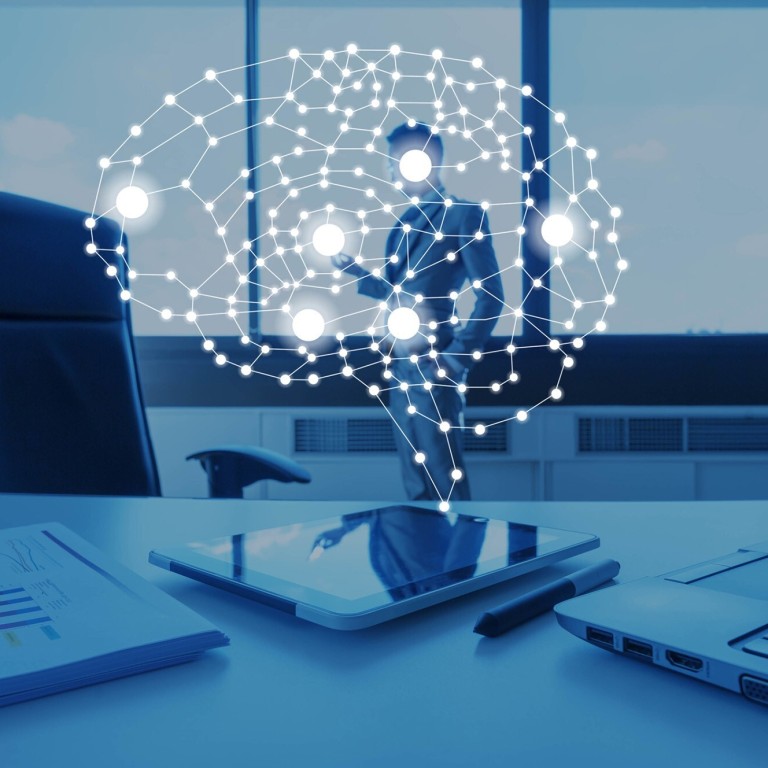
Machine learning systems, fair or biased, reflect our moral standards
- While machine learning systems and algorithms are theoretically more objective than humans, this does not necessarily result in different or fairer results
- There needs to be a concerted effort by regulators, governments and practitioners to ensure the technology stems inequities rather than perpetuates them
Discrimination in the real world is an ugly reality we have yet to change despite many years of effort. And recent events have made it clear that we have a long, long way to go.
Some companies and governments have turned to automation and algorithms in a bid to remove the human bias that leads to discrimination. However, while machine learning (ML) systems and algorithms are theoretically more objective than humans, the ability to apply the same decision structure unwaveringly does not necessarily result in different or fairer results.
Why is that? Given humans are their progenitors, bias is often built into these ML systems, leading to the same discrimination they were created to avoid. Recently, concerns have emerged that stem from the growing use of these ML systems, when unfiltered for bias, in such areas that could impact human rights and financial inclusion.
Today, ML algorithms are used in hiring, which could be biased by historical pay divides by gender; in parole profiling systems, impacted by historical trends of racial or geography-linked crime rates; and credit decisions, influenced by the economic status of a consumer segment that may have a racial tilt.
These biases stem from flaws in current processes which in turn, colour the training or modelling data set. This can then result in discrimination, and with the growing use of these systems at scale, existing social biases can be further amplified.
Computer, your biases are showing
How then can we filter out built-in biases and discrimination within these ML systems and make them “fairer” across every segment of society?
Let’s start by understanding what we mean by discrimination. Today, various forms of discrimination in making decisions about consumers have been identified and are subject to regulatory scrutiny. These include:
- Direct discrimination: when specific sensitive attributes like race, national origin, religion, sex, family status, disability, marital status or age are used to differentiate treatment.
- Indirect discrimination: when sensitive attributes are indirectly identified and used in the decision model. A typical example would be an attribute like postcode, which, while not categorised as a protected attribute, may still have a racial, religious and/or socio-economic concentration.
- Statistical discrimination: when decision-makers use average group statistics to judge an individual of the group, with racial profiling as a typical example.
- Systemic discrimination: policies or customs that are a part of the culture or structure of an organisation that may perpetuate discrimination against certain population subgroups, gender or racial stereotypes.
Too white, too male: scientist stakes out inclusive future for AI
ML systems learn from past decisions or from other feedback loops that are set up based on current decision agents, typically human. Consequently, they inherit human biases.
An ML algorithm typically optimises based on a finite data set. It is, therefore, subject to bias because an algorithm works on assumptions or classifiers. These assumptions and classifiers are necessary as without them, the algorithm would produce no better than random results. Unfortunately, this also means that the decision and outcomes from these algorithms can consequently be unfair and discriminatory.
Discrimination in the real world is an ugly reality we have yet to change despite many years of effort. And recent events have made it clear that we have a long, long way to go.
When evaluating fairness within ML systems, the metrics fall into two distinct categories – group and individual fairness. Group fairness is founded on the fair treatment of groups to ensure that members of all segments receive a fair share of the beneficial outcome(s). Individual fairness focuses on similar outcomes for similar individuals.
The commonly used group fairness metrics are:
- Demographic parity: acceptance rates are tracked across demographic profiles seen as disadvantaged to get them on par with the relatively advantaged segment. The fairness metric is achieved when the model’s classification is independent of the sensitive attribute(s).
- Equalised odds: no matter whether an applicant is part of an advantaged or disadvantaged segment, so long as they qualify, they are equally likely to get approved. If they do not qualify, they are equally likely to get rejected.
- Predictive (rate) parity: the fairness metric is met when the acceptance rate of a given classifier, e.g. race, is equivalent for each subgroup. This is also referred to as predictive parity.
The most commonly used individual fairness metrics are:
- Fairness through unawareness: this approach excludes sensitive attributes that lead to direct discrimination from the modelling data. While relatively easy to use, a key flaw is the availability of a multitude of correlated features that could indicate sensitive attributes in a typical ML model.
- Individual fairness or fairness through awareness: the fairness metric is reached when two applicants with a similar profile have the same probability of being approved.
- Counterfactual fairness: when a classifier produces the same result for one individual as it does for another identical individual, except for one or more sensitive attributes.
This gives us a starting point to measure fairness but there simply is no one-size-fits-all solution. It is impossible to satisfy all of the fairness constraints at once except in certain special cases. The fairness metric, therefore, needs to take into account the use case and the population distribution.
There needs to be a concerted effort by regulators, governments and practitioners to make sure that these algorithms and systems reflect the best in us, which helps to stem inequities, instead of the worst in us, which perpetuates them.
Recently, a lot of progress has been made to remove bias from ML through a variety of techniques. These include pre-processing, which focuses on the primary source of bias data; and post-processing, or altering the decision of the models to get to fair outcomes. Interestingly, a lot of academic research is currently centred around a third approach, which focuses on introducing a fairness definition into the model training process.
The decisions made through ML reflect our moral standards. There needs to be a concerted effort by regulators, governments and practitioners to make sure that these algorithms and systems reflect the best in us, which helps to stem inequities, instead of the worst in us, which perpetuates them. Only then can we hope to move towards a fairer world.
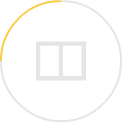